MARS (MetAbolomics ReSearch) is a vendor neutral desktop application software endowed with a Graphical User Interface (GUI) specifically developed for untargeted and semi-targeted LC-MS-based metabolomics and exposomics.
Differently form Lipostar, which was specifically designed for LC-MS based lipidomics with dedicated tools and workflows, MARS provides more general algorithms and investigation tools.
MARS fully covers all the steps required in LC-MS based untargeted and semitargeted metabolomics and exposomics analysis: instrument data conversion and processing, peak detection, statistical analysis, automated MS and/or MS/MS-based metabolite annotation, quantification, and biopathway analysis. Unique features have been developed in the software to improve annotation accuracy, including customizable identification of multiple adducts, automated in-source fragmentation detection, and in-silico MS/MS spectrum validation. Additionally, two MARS databases for exposomics (nitrosamines) and phytomics applications are available upon request.
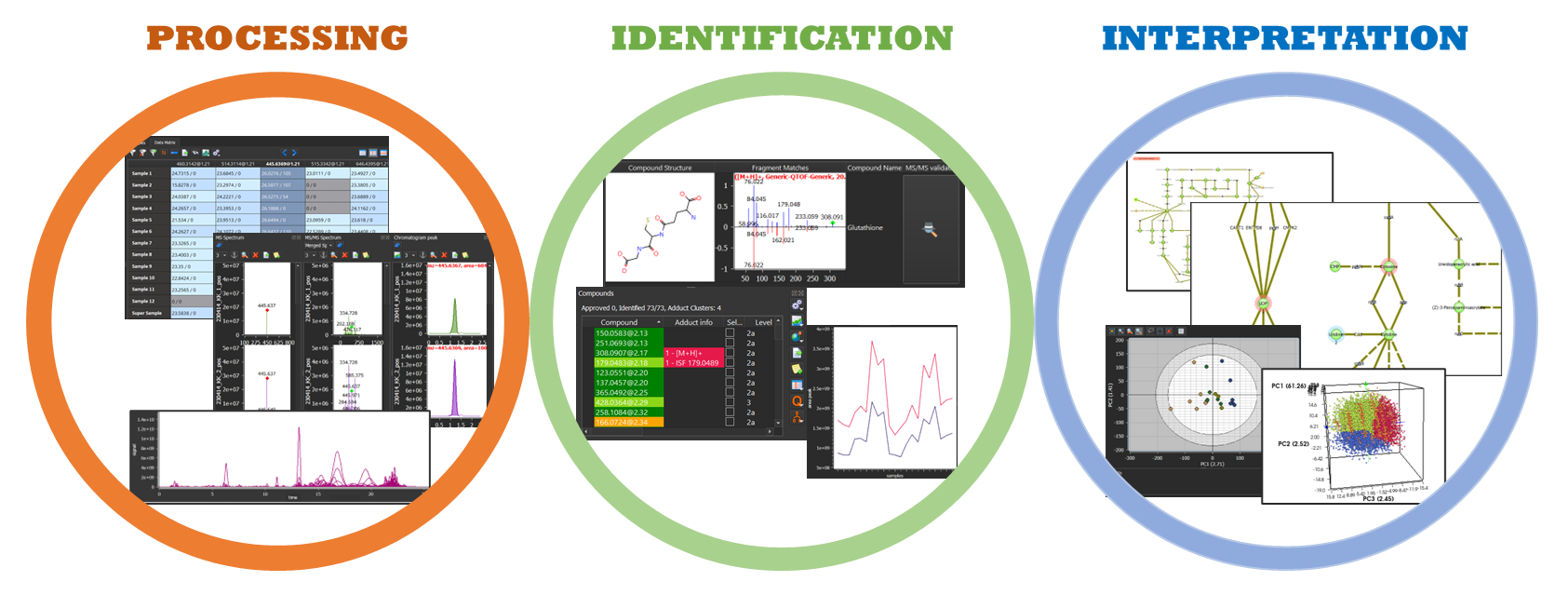
Key features
Database generation
- The MARS DB Manager module allows to generate customized databases based on internal data as well as automatically import data from The Human Metabolome Database (HMDB), MassBank of North America (MoNA), and Microbial Metabolites Database (MiMe). As already mentioned, two MARS databases for exposomics (nitrosamines) and phytomics applications are available upon request.
Data processing
Specific data processing algorithm:
- Baseline and noise reduction
- Peak extraction
- Peak smoothing (Statistical Deconvolution Algorithm or Savitzky-Golay)
- Signal-to-noise ratio
- Retention time (RT) correction
- Alignment
- Deisotoping
- Gap-filler (optional algorithm to reduce missing values in the data matrix)
A new peak detection algorithm for the processing of ion mobility spectrometry (IMS) data (IMS data are currently supported for Agilent, Waters, and Bruker).
Data matrix refinement
Several tools for data matrix refinement:
- Filters (e.g., blank subtraction, frequency filter, etc)
- Normalization by metadata (e.g., cell count, volume, weight)
- Normalization by analysis-related data (e.g., standards, total Area, QC, etc)
- Averaging over all replicates
- Merging of positive and negative data matrices
- Adduct clustering
Statistical analysis tool
MARS provides different analysis to investigate your data:
- Fold-change analysis
- Univariate statistical analysis (e.g., ANOVA)
- Principal Component Analysis (PCA)
- Consensus PCA
- Partial Least Squares regression (PLS)
- Partial Least Squares-Discriminant Analysis (PLS-DA)
- Orthogonal Partial Least Squares (O-PLS)
- Orthogonal Partial Least Squares-Discriminant Analysis (O-PLS-DA)
- Linear Discriminant Analysis (LDA)
Trend Analysis
An hypothesis-driven approach based on Pearson correlation coefficient or hypothesis-free cluster analysis (K-means and Bisecting K-means) are supported in MARS to extract trends of interest among samples.
Metabolite Identification
A flexible approach for metabolite identification is provided in MARS. It includes:
- A spectral matching approach for species included in the database (RT or CCS values, when available, can be used to improve the annotation accuracy)
- High-throughput approaches to detect other adducts and in-source fragmentations
- A MS/MS validator tool to re-check spectral matching assignation
- Clustering algorithm for adducts and in-source fragments of a same metabolite
- Tool for stable isotope labelling studies
- A score and a level-based classification as index of identification accuracy
- Preliminary search of xenobiotic metabolites
Quantification
Specific functionalities are provided in MARS for relative and absolute quantification using internal and/or external standards.
Pathway Analysis
MARS includes a collection of 20 metabolic pathways obtained by integrating data from different reference sources (KEGG metabolic network and PathBank linked to HMDB) and literature. The software also supports the projection of the identification results on metabolic pathways for functional analysis. The metabolics pathways available in MARS are:
- AAA biosynthesis
- Alanine aspartate and glutamate metabolism
- Arginine and proline metabolism
- Arginine biosynthesis
- Cysteine and methionine metabolism
- Glycolysis and gluconeogenesis
- GSH metabolism
- Histidine metabolism
- Lysine biosynthesis
- Lysine degradation
- N-glycan biosynthesis
- Pentose phosphate pathway
- Phenylalanine metabolism
- Purine pathway
- Pyrimidine metabolism
- TCA cycle
- Tryptophan metabolism
- Tyrosine metabolism
- Valine, leucine and isoleucine biosynthesis
- Valine, leucine and isoleucine degradation
Data support
- MARS supports the import of LC-MS and LC-MS/MS data from the following mass-spec vendors:
- Agilent(*.d): AutoMS and full scan at multiple energies of collision (All Ions).
- Waters(*.raw): MSe, HDMSe, DDA, and MSMS, SONAR.
- Thermo(*.RAW): Ion-Trap and Orbitrap, Exactive, Q-Exactive, DDA and AIF.
- Sciex(*.wiff): SWATH and IDA.
- Bruker(*.d): QTof, FT-ICR, TIMS-TOF data dependent scan.
- Shimadzu(*.lcd): QTof.
- Ion mobility spectrometry (IMS) data are supported for Agilent(*.d), Waters(*.raw), and Bruker(*.d).
- Agilent(*.d), Waters(*.raw), and Shimadzu(*.lcd) files can be directly imported.
- Thermo(*.RAW), Bruker(*.d), and Sciex(*.swiff) files require the use of a converter downloadable from the instrument site.
Requirements
Thermo requirements:
- MSFileReader 3.1 SP3
- MSFileReader 3.1 SP4
Bruker requirements:
- CompassXtract package
Sciex requirements:
- MMS+Wiff+Access+Patch+2-win64.exe
Additional libraries required are listed in the software manual
System requirement and installation
MARS can be installed only on a 64bit Windows operating system.
MARS Training documents – Version 1.0.3
- Tutorial_MARS_01 Select your style before you begin
- Tutorial_MARS_02 The MARS DB manager
- Tutorial_MARS_03 Generating a fully labelled database
- Tutorial_MARS_04 Data processing and statistical analysis
- Tutorial_MARS_05 Metabolite identification and annotation levels
- Tutorial_MARS_06 Exploring metabolite pathways
- Tutorial_MARS_07 The trend analysis: filtering global profiling data by anticipated trends
- Tutorial_MARS_08 Normalization
- Tutorial_MARS_09 Metabolite quantification
- Tutorial_MARS_10 Grouping adducts and in-source fragments
- Tutorial_MARS_11 Data export and report generation
- Tutorial_MARS_12 MARS for N-nitrosamine detection
Articles:
-
MARS: A Multipurpose Software for Untargeted LC–MS-Based Metabolomics and Exposomics
- January 18, 2024. Laura Goracci*, Paolo Tiberi, Stefano Di Bona, Stefano Bonciarelli, Giovanna Ilaria Passeri, Marta Piroddi, Simone Moretti, Claudia Volpi, Ismael Zamora and Gabriele Cruciani
Database Information
- File name: db_PHYTO_240531
- Number of compounds: 29,750
- Classification: 10 main classes and 70 sub-classes
- Number of MS/MS spectra: 10,826
- Type of MS/MS spectra: rule-based fragmentation (virtual)
- Details:
- The database contains the structure, formula, exact mass, MS1 of 29,750 phytochemicals and 10,826 MS2 information.
- The dataset of 29,750 phytochemicals was collected from four databases (KEGG, LipidMaps, HMDB, and PhenolExplorer) and classified into 10 main classes and 70 subclasses.
- The MS2 rule-based fragmentation was applied to different subclasses of phytochemicals. In particular, it has been adopted for the classes of flavonoids, alkaloids, and phenolic acids and derivatives.
- Nomenclature assignation: An identification code (ID) consisting of an alphanumeric string of four and different numbers is assigned to each phytochemical in the database. In addition, a common name is associated with each compound based on the common nomenclature used in KEGG, LipidMaps, HMDB, and PhenolExplorer databases.
- Fragmentation rules: Fragmentation rules were coded from experimental fragmentation of phytochemicals collected from literature and from in-house acquired data.
Database Information
- File name: db_nitrosamines_20240531
- Number of compounds: 28,024
- Classification: two classes (linear nitrosamines, cyclic nitrosamines)
- Number of MS/MS spectra: 28,024
- Type of MS/MS spectra: rule-based fragmentation (virtual)
- Details:
- The database contains the structure, formula, exact mass, MS1, and MS2 information for 28,024 nitrosamines. Both linear and cyclic nitrosamines are included in the database. In particular, the linear nitrosamines included in the database are 27,856, while the cyclic nitrosamines are 168.
- Nitrosamines compounds derive from different data sources:
- nitrosamines reported by Regulatory Agencies (e.g., EMA and FDA);
- nitrosamines distributed by commercial suppliers;
- nitrosamines generated in-silico.
- Table 1. Number of entries included in the database from the different sources
- Regulatory Agencies: 141
- Commercial suppliers: 209
- In silico generation: 27,674
- Total number: 28,024
- Nomenclature assignation:
- An identification code (ID) consisting of an alphanumeric string of two letters and 7 numbers (i.e., NA0000001, NA0000002, NA0000003, etc.) is assigned to each nitrosamine in the database. In addition, a common name is associated with each compound.
- The schematic common name for linear nitrosamines is NO(N-X/N-Y) where X and Y can represent:
- aliphatic chains bonded to the N-nitroso group. Aliphatic chains are represented in the common name as “C:DB” where C is the number of carbon and DB is the number of double bonds in the chains. Example for N-nethylethylamine (NMEA), common name: NO(N-1:0/N-2:0).
- substituent different from aliphatic chains bonded to the N-nitroso group. This kind of substituents are represented in the common name with an alphanumeric string. Example for N-nitrosodiphenylamine (NDPhA), common name: NO(N-Ph/N-Ph).
- In contrast, the schematic common name for cyclic nitrosamines is NO(C-Z), where Z is an alpha-numeric string. Example for N-nitrosomorpholine (NMOR), common name: NO(C-MOR); N-nitrosopiperidine (NPIP), common name: NO(C-PIP); and N-nitrosopyrrolidine (NPYR), common name: NO(C-PYR).
- Fragmentation rules: Fragmentation rules were coded from experimental fragmentation of nitrosamines collected from literature and from in-house acquired data.
Is the chromatogram visualized in the “Sample” tab of “Data Analysis” page the sum of XIC of all compounds in a specific sample?
The chromatogram visualized by selecting one sample in the “Sample” tab of the “Data Analysis”page is the sum of the reconstructed chromatographic peaks of all the chemical features detected in the selected sample.
How to understand which signals are rescued by gap filler algorithm?
In the data-matrix the cells filled by the gap-filler algorithm are highlighted by light-blue color for positive acquired data and light-red color for negative acquisition. Chemical features with signal above the threshold for processing are instead shown in blue for positive acquired data and red color for negative acquisition.
Can MARS show the P value obtained after ANOVA/fold change analysis?
P values are shown at the end of the process in the table containing the results.
Can MARS show increasing or decreasing metabolites that populate a note with different colors in the pathway maps?
The user can connect the identification results to metabolic pathways and compare metabolites that increase, or decrease based on label comparison. For that you can refer to Tutorial number 6.4.
What is the difference between p-value and corr p-value in the ANOVA/fold change analysis? Is the provided p-value the Anova P value?
P-values are Anova p-values. Corr p-values are “adjusted or corrected” p-values based on Benjamini-Hochberg procedure
In the identification tab, for the Compounds section, there is a High and a p-High value shown. What are these values and what do they reference?
High means number of identifications with high confidence. P-high (abbreviation of promoted-high) means number of identifications promoted high. The p-high identifications are those found by MARS during the second run of the identification. In this second run, MARS searches for the other adducts included by the user in the identification method ([M+H]+ and [M-H]- are investigated during the first run of the identification) and for in-source fragmentations. More details are reported in MARS publication. (DOI: 10.1021/acs.analchem.3c03620).
What should be the score associated with high confidence identifications?
Both 4 and 3 stars of confidence are considered high. Therefore, to have a high confidence identification the overall score associated with it should be greater than 60.
When running the MS/MS Validator, there is a “Save” icon that appears once it’s done. What does the save do, and where does it save the information? It looks like it creates a theoretical fragment entry for the match?
The “Save” icon that appears at the end of the MS/MS validator running allows updating the theoretical fragment ions collected in the DB connected to the MARS session for that given compound. The fragment ions that will be saved on the database are only those found by the MS/MS validator. The fragment ions previously collected, will be overwritten.
How can I create my own MSMS spectral database? How can I populate the metadata?
To generate a library from in-house acquired data, related information must be imported using a .csv file into the DB Manager. The .csv contains different information on the compound/s to be imported (i.e., Id, Common Name, Formula, Classification, RT, Adducts – positive and negative ionization adducts are allowed, SMILES, and the name of the instrument data files from which to import the MS and MS/MS information for each specified compound). The step-by-step description of the workflow to generate an in-house MSMS spectral database into the DB Manager is reported in Tutorial 2.2 (pag13).
When doing an identification using the Merged MSMS functionality, I only see one matched spectrum. Is this normal? If not, how is the Merged feature supposed to work?
The merged MSMS functionality in the identification method allows to carry out the identification process using as reference for the spectral matching approach the merged spectrum of those included in the database for each single compound. Therefore, it is normal to have only one matched spectrum.
What are the different instrument type definitions for the identification method? How is this information applied to custom databases? Is there a way to see the instrument type in MARS DB Manager? Is there a way to filter based on instrument type?
MARS allows the generation of databases for identification purposes by importing the experimental MSMS spectra collected in the HMDB or MoNa repository. Generally, the information of instruments used to acquire the experimental spectrum is reported. Therefore, in MARS the user can perform the identification using different options:
- Use each spectrum included in the database to perform the identification (experimental MSMS vs all the MSMS spectra in the database).
- Use only the MSMS spectra in the database acquired with a user-defined collision energy to perform the identification.
- Use only the MSMS spectra in the database acquired with a specific instrument type that the user can specify in the identification method to perform the identification.
- Use the merged database described above to perform the identification.
Is it possible to filter non-identified data – so we can specifically review those compounds as a simple data matrix for further interrogation?
The user can filter non-identified data by clicking on the “operation” icon (gear icon) in the “Result” tab of the “Identification” page and then on “Filter results”. In the new window, the user needs to select “Compound Class Filter” and then “Not Identified” in the drop-down menu.